The Discovery: Two Sigma PhD Symposium
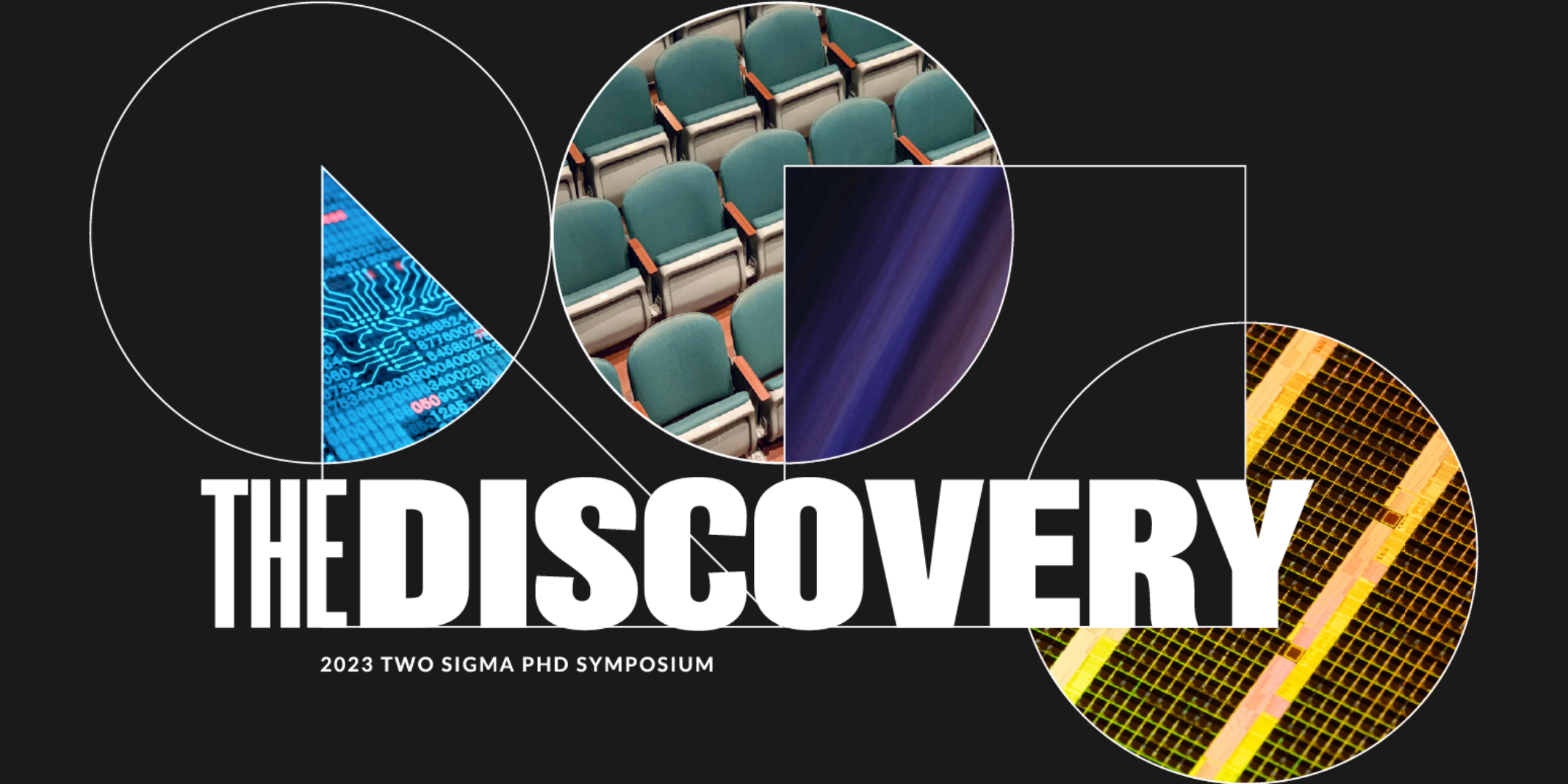
An invitation only afternoon of knowledge sharing and innovation.
Two Sigma uses third-party advertising and advertising analytics cookies that allow us and our partners to serve your more relevant advertisements across platforms. You may accept or decline our use of these kinds of cookies by selecting “accept” or “decline” below. For more information about our privacy practices, please visit our Cookies Policy here.
An invitation only afternoon of knowledge sharing and innovation.
The Discovery, Two Sigma’s PhD Symposium, brought together the top PhD students from across the United States to exchange ideas with fellow doctoral students, distinguished professors, and Two Sigma researchers at our Soho headquarters. This invite-only event offers doctoral students with the unique opportunity to share and solicit feedback on their current research.
Participants stayed at the nearby Roxy Hotel in TriBeCA.
Thursday, June 15th
Two Sigma
101 Avenue of the Americas
New York, NY, 10013
The visiting doctoral students attended a breakfast hosted at Two Sigma's Soho headquarters where they had the opportunity to network with your fellow presenters and Two Sigma researchers.
Ken Baron, Managing Director & Quantitative Researcher, welcomed the doctoral students to Two Sigma and introduced the first speaker of the day, a distinguished professor who shared their current research on neural networks.
Throughout the afternoon, the visiting doctoral students shared an overview of their research areas on topics spanning physics, applied mathematics, computer science, statistics, and econometrics. Following their presentations, the students fielded questions from Two Sigma's researchers and their peers.
A reception on Two Sigma's rooftop terrace followed the presentations and talks with distinguished professors. Participants enjoyed refreshments, including a color changing mocktail and mini ice cream cones made-to-order in liquid nitrogen.
Two Sigma’s Academic Partnerships program aims to support and recognize outstanding students and educators who share our passion for learning and seeking a deeper understanding of the world around us.
The Academic Partnerships program offers universities, research labs, professors, and students many ways to connect with Two Sigma. Our mission is to foster inclusive academic communities where academics of all backgrounds feel empowered to expand frontiers in STEM.
Cornell University
Physics
Machine Learning in Physics Education Research: Toward making trustworthy claims with machine coded data
As interest increases in using natural language processing methods (“machine coding”) to supplant labor-intensive human coding of student survey responses, the physics education research community needs methods to determine the accuracy and reliability of machine coding. Existing methods do not allow researchers and educators to trust an algorithm without a time-consuming manual check. I demonstrate how both statistical and systematic uncertainty of machine coding can be quantified. Furthermore, I will show how uncertainty is dependent on training set characteristics and test set characteristics, and I present methodology that allows researchers and educators to use their scientific skills to gain trust in an algorithm.
Stanford University
Physics
In Search of No Neutrinos: The nEXO Experiment and Detector Calibration
The imbalance between matter and antimatter in the universe remains one of the most perplexing mysteries of modern physics. One possible explanation suggests that this imbalance originates in the neutrino sector. According to this hypothesis, a neutrino is its own antiparticle, defying the usual matter-antimatter distinction. If this were true, a rare phenomenon could be observed: the mutual annihilation of two antineutrinos in a double beta decay. This occurrence would leave a distinctive signature that could be detected. In this talk I will introduce nEXO, an experiment designed to detect this phenomenon, and describe my work developing a calibration scheme for the planned detector.
Yale University
Applied Mathematics
Riemannian Geometry in Machine Learning
Although machine learning researchers have introduced a plethora of useful constructions for learning over Euclidean space, numerous types of data in various applications benefit from, if not necessitate, a non-Euclidean treatment. For example, consider representing the dynamics of segments in time series data by their covariance matrices, which lie on the manifold of symmetric positive definite (SPD) matrices. In contexts where data points lie on non-trivial Riemannian manifolds, one must devise methods to properly learn over such data while respecting manifold structure. To this end, I have written the ICML 2020 paper “Differentiating through the Fréchet Mean” [1], and am in the process of writing a new paper, “Riemannian Residual Neural Networks.” I will present both of these papers in light of the aforementioned motivation.
References
[1] Aaron Lou, Isay Katsman, Qingxuan Jiang, Serge Belongie, Ser-Nam Lim, and Christopher De Sa. Differentiating through the fréchet mean. In International Conference on Machine Learning, 2020.
Stanford University
Computer Science
Fairness and Robustness with Missing Information
The reliability of machine learning systems critically assumes that the associations between features and labels remain similar between training and test distributions. However, unmeasured variables, such as confounders, break this assumption—useful correlations between features and labels at training time can become useless or even harmful at test time. For example, high obesity is generally predictive for heart disease, but this relation may not hold for smokers who generally have lower rates of obesity and higher rates of heart disease. We present a framework for making models robust to spurious correlations by leveraging humans’ common sense knowledge of causality. Specifically, we use human annotation to augment each training example with a potential unmeasured variable (i.e. an underweight patient with heart disease may be a smoker), reducing the problem to a covariate shift problem. We then introduce a new distributionally robust optimization objective over unmeasured variables (UV-DRO) to control the worst-case loss over possible test-time shifts. Empirically, we show improvements of 5-10% on a digit recognition task confounded by rotation, and 1.5-5% on the task of analyzing NYPD Police Stops confounded by location.
Harvard University
Computer Science
Online Reinforcement Learning Algorithms for Digital Interventions
We describe the development of an online reinforcement learning (RL) algorithm for use in optimizing the delivery of mobile-based prompts to encourage oral hygiene behaviors. One of the main challenges in developing such an algorithm is ensuring that the algorithm considers the impact of the current action on the effectiveness of future actions (i.e., delayed effects), especially when the algorithm has been made simple in order to run stably and autonomously in a constrained, real-world setting (i.e., highly noisy, sparse data). We address this challenge by designing a quality reward which maximizes the desired health outcome (i.e., high-quality brushing) while minimizing user burden. We also highlight a procedure for optimizing the hyperparameters of the reward by building a simulation environment test bed and evaluating
candidates using the test bed. The RL algorithm discussed in this paper is currently deployed in Oralytics, an oral self-care app that provides behavioral strategies to boost patient engagement in oral hygiene practices.
University of North Carolina
Statistics
Evaluating the Robustness of Parametric Maximum Likelihood Estimation for Handling Randomly Right Censored Covariates
Cognitive dysfunction is a symptom of Huntington’s disease and can serve as an early marker for evaluating treatments intended at delaying the disease. Understanding cognitive dysfunction as a function of age at clinical diagnosis is a prerequisite for their use in clinical trials, but this remains challenging because age at clinical diagnosis is not always observed; patients drop out or the study ends, resulting in a censored value for age at clinical diagnosis. Parametric maximum likelihood estimation is a flexible estimation method that accounts for randomly right censored covariates, such as age at clinical diagnosis. Still, robustness depends on the choice of the parametric distribution, and potentially the choice of the generalized linear model. In this study, we evaluate robustness aspects of parametric maximum likelihood estimation against misspecification of the conditional distribution of a right censored covariate. We evaluate robustness in linear and logistic regression to reveal which model is prone to bias under misspecification, which may help Huntington’s disease researchers leverage the potential for bias. Simulation results show that logistic regression, when compared to linear regression, achieves lower bias, higher accuracy in standard error estimation, and higher coverage. We apply the parametric maximum likelihood estimator to the Neurobiological Predictors of Huntington’s Disease study and analyze a measure of cognitive dysfunction (i.e., Symbol Digit Modalities Test). Results show that parametric maximum likelihood estimation results in comparable but more efficient estimates when compared to the complete case estimator.
Carnegie Mellon University
Econometrics/Finance
Equity Compensation and Firm Value
In many industries, equity compensation ties a substantial portion of non-executive employee income to firm performance. We hypothesize shocks affecting equity-paying firms are amplified due to their simultaneous impact on firm profitability and employee turnover. To measure this effect, we study the forfeiture rates of unvested equity grants against employee returns, and we find that a 10% increase in returns reduces forfeiture rates by 1.4%. To understand the role of equity sensitivity on firm value, we incorporate our hypothesis into an investment model and find that the cross-sectional average and standard deviation of firm value are weakly increasing in the equity share. This amplification effect implies a channel by which a firm’s capital structure is relevant to its value, in contrast to the Miller-Modigliani Theorem of capital structure irrelevance.
University of California, Berkeley
Statistics
A Generic Approach for Reproducible Model Distillation
Model distillation has been a popular method for producing interpretable machine learning. It uses an interpretable “student” model to mimic the predictions made by the black box “teacher” model. However, when the student model is sensitive to the variability of the data sets used for training even when keeping the teacher fixed, the corresponded interpretation is not reliable. Existing strategies stabilize model distillation by checking whether a large enough corpus of pseudo-data is generated to reliably reproduce student models, but methods to do so have so far been developed for a specific student model. In this paper, we develop a generic approach for stable model distillation based on central limit theorem for the average loss. We start with a collection of candidate student models and search for candidates that reasonably agree with the teacher. Then we construct a multiple testing framework to select a corpus size such that the consistent student model would be selected under different pseudo samples. We demonstrate the application of our proposed approach on three commonly used intelligible models: decision trees, falling rule lists and symbolic regression. Finally, we conduct simulation experiments on
Mammographic Mass and Breast Cancer datasets and illustrate the testing procedure throughout a theoretical analysis with Markov process.