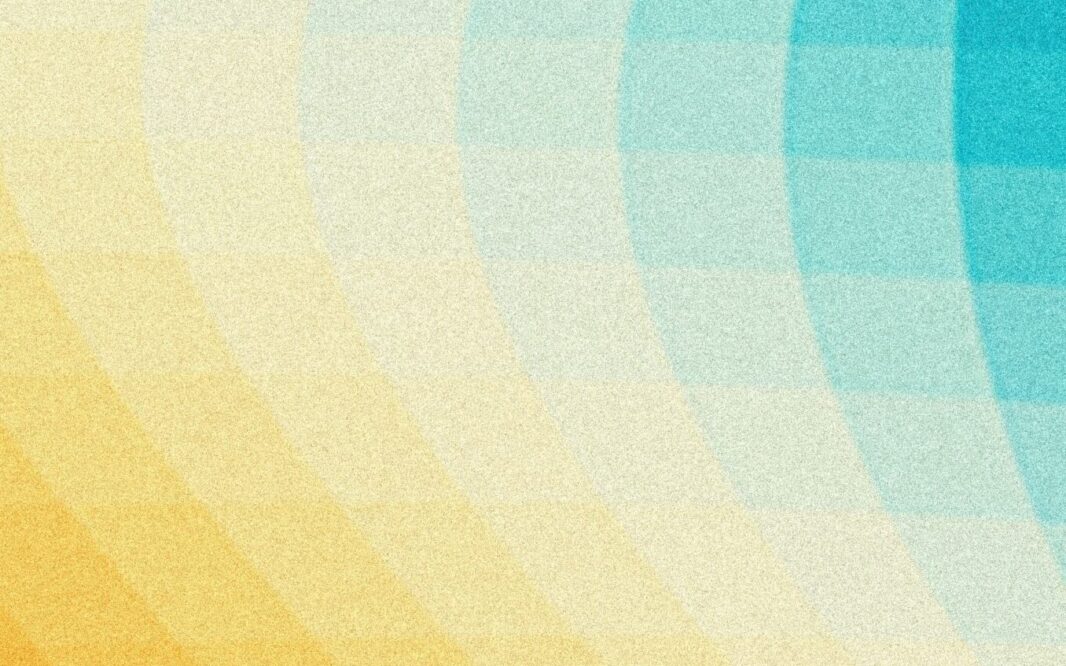
NeurIPS 2023: Our Favorite Papers on LLMs, Statistical Learning, and More
Two Sigma researchers highlight some of the most captivating papers and presentations on machine learning from NeurIPS 2023.
Two Sigma researchers highlight some of the most captivating papers and presentations on machine learning from NeurIPS 2023.
Drew Conway, Head of Data Science, Private Investments, spoke with Institutional Real Estate, Inc. about how data science, engineering, and AI can be applied to real estate investing.
Hypothesizing is nice, but gathering evidence is better.